Claude 3.5 Sonnet Multi-Modal Learning.In the ever-evolving landscape of artificial intelligence, Claude 3.5 Sonnet stands out as a groundbreaking model that pushes the boundaries of what AI can achieve. At the heart of its extraordinary capabilities lies a sophisticated multi-modal learning system that enables it to process and understand information across various formats. This article delves deep into the world of Claude 3.5 Sonnet’s multi-modal learning, exploring how it works, its applications, and the profound impact it’s having on the field of AI.
Understanding Multi-Modal Learning in AI
Before we dive into the specifics of Claude 3.5 Sonnet, it’s crucial to understand what multi-modal learning means in the context of artificial intelligence. Traditional AI models often specialize in processing one type of data, such as text or images. Multi-modal learning, however, refers to the ability of an AI system to integrate and process information from multiple sources or formats simultaneously.
This approach to AI learning mirrors the way humans perceive and understand the world around them. We don’t rely on a single sense to gather information; instead, we combine visual, auditory, and other sensory inputs to form a comprehensive understanding of our environment. Claude 3.5 Sonnet brings this human-like perception to the world of AI, opening up new possibilities for more nuanced and context-aware artificial intelligence.
The Architecture of Claude 3.5 Sonnet’s Multi-Modal System
At its core, Claude 3.5 Sonnet’s multi-modal learning system is built on a sophisticated neural network architecture that allows for the seamless integration of various data types. This architecture is designed to process and understand multiple input modalities, including but not limited to:
- Text: Natural language in various forms, from short queries to long-form content.
- Images: Static visual data, including photographs, diagrams, and artwork.
- Audio: Sound files, including speech and music.
- Video: Moving visual data with accompanying audio.
- Structured Data: Tabular data, graphs, and other organized information formats.
The key to Claude 3.5 Sonnet’s success lies in its ability to not just process these different types of data independently, but to integrate them into a unified understanding. This is achieved through a complex system of neural pathways that allow information from different modalities to interact and inform each other.
The Fusion Layer: Where Magic Happens
One of the most critical components of Claude 3.5 Sonnet’s multi-modal architecture is the fusion layer. This layer acts as a bridge between the different modalities, allowing the model to combine information from various sources in a meaningful way.
The fusion layer employs advanced attention mechanisms that enable Claude 3.5 Sonnet to focus on the most relevant aspects of each input modality. For example, when analyzing a news article with accompanying images, the fusion layer helps the model determine which parts of the text correspond to which elements of the images, creating a cohesive understanding of the content.
This ability to fuse information from multiple sources allows Claude 3.5 Sonnet to generate more accurate and contextually relevant responses, making it an invaluable tool for a wide range of applications.
The Training Process: How Claude 3.5 Sonnet Learns
The multi-modal capabilities of Claude 3.5 Sonnet are the result of an intensive and carefully designed training process. This process involves exposing the model to vast amounts of diverse data across different modalities, allowing it to learn the intricate relationships between various types of information.
Diverse Dataset Curation
The first step in training Claude 3.5 Sonnet’s multi-modal system involves curating a diverse and comprehensive dataset. This dataset includes:
- Millions of text documents spanning various topics and writing styles
- A vast collection of images, ranging from photographs to abstract art
- Audio files, including spoken language in multiple dialects and accents
- Video content covering a wide range of subjects and styles
- Structured data from various domains, such as scientific research and financial reports
The diversity of this dataset is crucial, as it allows Claude 3.5 Sonnet to develop a broad understanding of different types of information and how they relate to each other.
Cross-Modal Learning Techniques
During the training process, Claude 3.5 Sonnet employs advanced cross-modal learning techniques. These techniques involve presenting the model with data from multiple modalities simultaneously and teaching it to identify relationships between different types of input.
For example, the model might be shown an image along with a textual description. Through repeated exposure to such paired data, Claude 3.5 Sonnet learns to associate visual elements with their textual descriptions, and vice versa. This cross-modal learning extends to all combinations of input types, allowing the model to develop a rich, interconnected understanding of various data formats.
Continual Learning and Adaptation
One of the most impressive aspects of Claude 3.5 Sonnet’s training process is its ability to engage in continual learning. Unlike some AI models that are trained once and then deployed, Claude 3.5 Sonnet is designed to continuously adapt and improve its understanding based on new information.
This continual learning process allows the model to stay up-to-date with evolving knowledge and to refine its multi-modal understanding over time. It also enables Claude 3.5 Sonnet to adapt to new types of data or novel combinations of modalities that it may encounter after its initial training.
Real-World Applications of Claude 3.5 Sonnet’s Multi-Modal Learning
The multi-modal capabilities of Claude 3.5 Sonnet open up a world of possibilities across various industries and applications. Let’s explore some of the most impactful ways this technology is being put to use:
Advanced Content Analysis and Creation
In the world of digital content, Claude 3.5 Sonnet’s multi-modal learning shines. The model can analyze articles, videos, and social media posts, understanding not just the text but also the accompanying images, audio, or video content. This comprehensive analysis allows for more accurate content categorization, sentiment analysis, and trend prediction.
Moreover, Claude 3.5 Sonnet can assist in content creation by generating text that is contextually relevant to given visual or audio inputs. For example, it can help create detailed product descriptions based on product images or generate video scripts that align perfectly with existing footage.
Enhanced Visual Question Answering
Visual Question Answering (VQA) is a field where Claude 3.5 Sonnet’s multi-modal capabilities truly excel. The model can analyze an image and accurately answer questions about its content, understanding both the visual elements and the nuances of the questions asked.
This capability has numerous applications, from assisting visually impaired individuals in understanding their surroundings to helping researchers quickly extract information from scientific images or diagrams.
Revolutionizing Medical Diagnosis
In the medical field, Claude 3.5 Sonnet’s multi-modal learning is proving to be a game-changer. The model can analyze medical images such as X-rays or MRIs alongside patient records and symptom descriptions. By integrating information from these various sources, Claude 3.5 Sonnet can assist healthcare professionals in making more accurate diagnoses and treatment recommendations.
The ability to process and understand multiple types of medical data simultaneously allows for a more holistic approach to patient care, potentially leading to improved outcomes and more personalized treatment plans.
Advancing Scientific Research
Scientists across various disciplines are leveraging Claude 3.5 Sonnet’s multi-modal capabilities to accelerate their research. The model can analyze scientific papers, experimental data, and visual representations simultaneously, helping researchers identify patterns and connections that might be missed by human analysis alone.
For example, in fields like astronomy or particle physics, Claude 3.5 Sonnet can analyze vast amounts of observational data alongside theoretical models, potentially leading to new discoveries or insights.
Enhancing Educational Tools
In the realm of education, Claude 3.5 Sonnet’s multi-modal learning is being used to create more engaging and effective learning tools. The model can understand and generate content across various formats, allowing for the creation of interactive learning experiences that cater to different learning styles.
For instance, an educational app powered by Claude 3.5 Sonnet could provide text-based explanations, visual aids, and even generate practice problems based on a student’s individual needs and learning progress.
The Ethical Implications of Multi-Modal AI
As with any advanced AI technology, the multi-modal capabilities of Claude 3.5 Sonnet raise important ethical considerations. While the potential benefits are immense, it’s crucial to address the potential risks and challenges associated with such powerful AI systems.
Privacy Concerns
One of the primary ethical concerns surrounding multi-modal AI is privacy. Claude 3.5 Sonnet’s ability to process and understand various types of data, including images and audio, raises questions about data protection and individual privacy rights. It’s essential that strict protocols are in place to ensure that personal information is protected and that the model is used in ways that respect user privacy.
Bias and Fairness
Another critical ethical consideration is the potential for bias in multi-modal AI systems. If the training data used to develop Claude 3.5 Sonnet is not sufficiently diverse or contains inherent biases, these biases could be reflected in the model’s outputs. This is particularly concerning given the model’s ability to influence decision-making across various fields, from healthcare to education.
Addressing this challenge requires ongoing efforts to ensure diversity and representation in training data, as well as regular audits of the model’s outputs to identify and correct any biases that may emerge.
Transparency and Explainability
The complexity of multi-modal AI systems like Claude 3.5 Sonnet can make it challenging to understand how the model arrives at its conclusions or recommendations. This lack of transparency can be problematic, especially in high-stakes applications like medical diagnosis or financial decision-making.
Efforts are underway to develop methods for explaining the decision-making processes of multi-modal AI systems, but this remains an active area of research and development.
The Future of Multi-Modal Learning in AI
As impressive as Claude 3.5 Sonnet’s multi-modal capabilities are, they represent just the beginning of what’s possible in this field. Looking to the future, we can anticipate several exciting developments:
Expanded Modalities
While Claude 3.5 Sonnet already processes a wide range of data types, future iterations may expand to include even more modalities. This could include tactile data, allowing the AI to understand and simulate physical sensations, or even olfactory data, enabling it to process information about scents and smells.
Enhanced Cross-Modal Understanding
Future developments in multi-modal AI are likely to focus on deepening the connections between different modalities. This could lead to AI systems that can translate concepts seamlessly between different formats, for example, generating a piece of music that perfectly captures the mood of a painting.
Real-Time Multi-Modal Processing
As processing power continues to increase, we may see multi-modal AI systems like Claude 3.5 Sonnet able to process and integrate information from multiple sources in real-time. This could enable applications like advanced augmented reality systems that can provide instant, context-aware information about the user’s environment.
Integration with Robotics
The multi-modal learning capabilities of AI systems like Claude 3.5 Sonnet have significant implications for the field of robotics. By integrating multi-modal AI with advanced robotics, we could see the development of robots that can interact with their environment in more human-like ways, understanding and responding to visual, auditory, and even tactile inputs.
Conclusion: The Transformative Power of Multi-Modal AI
Claude 3.5 Sonnet’s multi-modal learning capabilities represent a significant leap forward in the field of artificial intelligence. By mimicking the human ability to integrate information from multiple sources, this technology is opening up new possibilities across various industries and applications.
From revolutionizing healthcare diagnostics to enhancing scientific research, from creating more engaging educational tools to enabling more nuanced content analysis, the impact of multi-modal AI is far-reaching and profound.
However, as we continue to develop and deploy these powerful AI systems, it’s crucial that we remain mindful of the ethical implications and potential challenges. Ensuring privacy, addressing biases, and striving for transparency should be at the forefront of ongoing research and development efforts.
As we look to the future, the potential of multi-modal AI seems boundless. With continued advancements in this field, we can anticipate AI systems that are increasingly capable of understanding and interacting with the world in ways that are truly human-like.
Claude 3.5 Sonnet’s multi-modal learning capabilities are not just a technological achievement; they represent a fundamental shift in how we think about artificial intelligence. By breaking down the barriers between different types of data and enabling more holistic understanding, this technology is paving the way for a future where AI can be a more intuitive, context-aware, and ultimately more valuable tool in our quest to understand and improve the world around us.
As we stand on the brink of this new era in AI, one thing is clear: the journey of discovery and innovation in multi-modal learning is far from over. With each new development, we move closer to a world where AI can truly see, hear, and understand in ways that were once the stuff of science fiction. The future of AI is multi-modal, and with technologies like Claude 3.5 Sonnet leading the way, that future is looking brighter and more exciting than ever before.
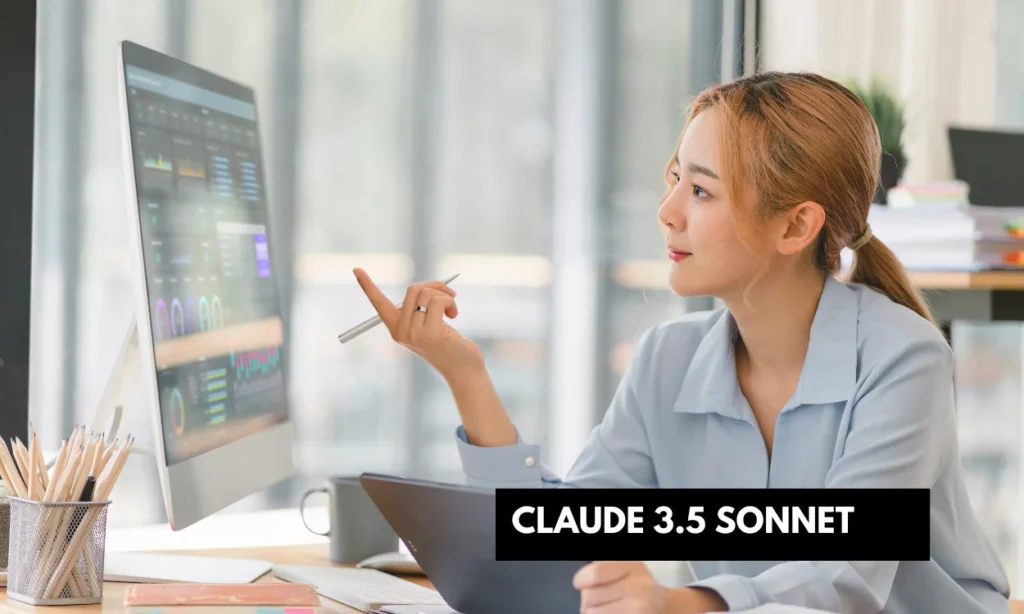
FAQs
What is Multi-Modal Learning in the context of Claude 3.5 Sonnet?
Multi-Modal Learning in Claude 3.5 Sonnet refers to the model’s capability to process and integrate information from various types of data inputs, such as text, images, and audio, to enhance its understanding and generate more contextually relevant responses.
How does Claude 3.5 Sonnet utilize Multi-Modal Learning?
Claude 3.5 Sonnet leverages Multi-Modal Learning by combining insights from different modalities (e.g., text and images) to provide richer and more nuanced outputs, improving its ability to understand complex queries and provide comprehensive responses.
What types of data can Claude 3.5 Sonnet process using Multi-Modal Learning?
Claude 3.5 Sonnet can process various types of data, including text, images, audio, and potentially video, integrating these modalities to enhance its overall understanding and response generation.
How does Multi-Modal Learning improve the model’s performance?
Multi-Modal Learning improves performance by allowing the model to draw on a more diverse set of information sources, which helps in understanding context more deeply, handling ambiguous queries better, and generating more accurate and relevant responses.
What are the benefits of integrating text and images in Claude 3.5 Sonnet?
Integrating text and images allows Claude 3.5 Sonnet to provide more contextually accurate responses, such as describing images in detail, answering questions about visual content, and enhancing understanding by correlating visual and textual information.